Mariana Castaneda-Guzman
IGC FELLOW | Global Change Center
Ph.D. Student • Fish and Wildlife Conservation
Advisors : Dr. Paul Angermeier and Dr. Emmanuel Frimpong
Research Interests: Data analytics, remote sensing, ecological niche modeling, climate change, biodiversity conservation
marianacg@vt.edu • CV
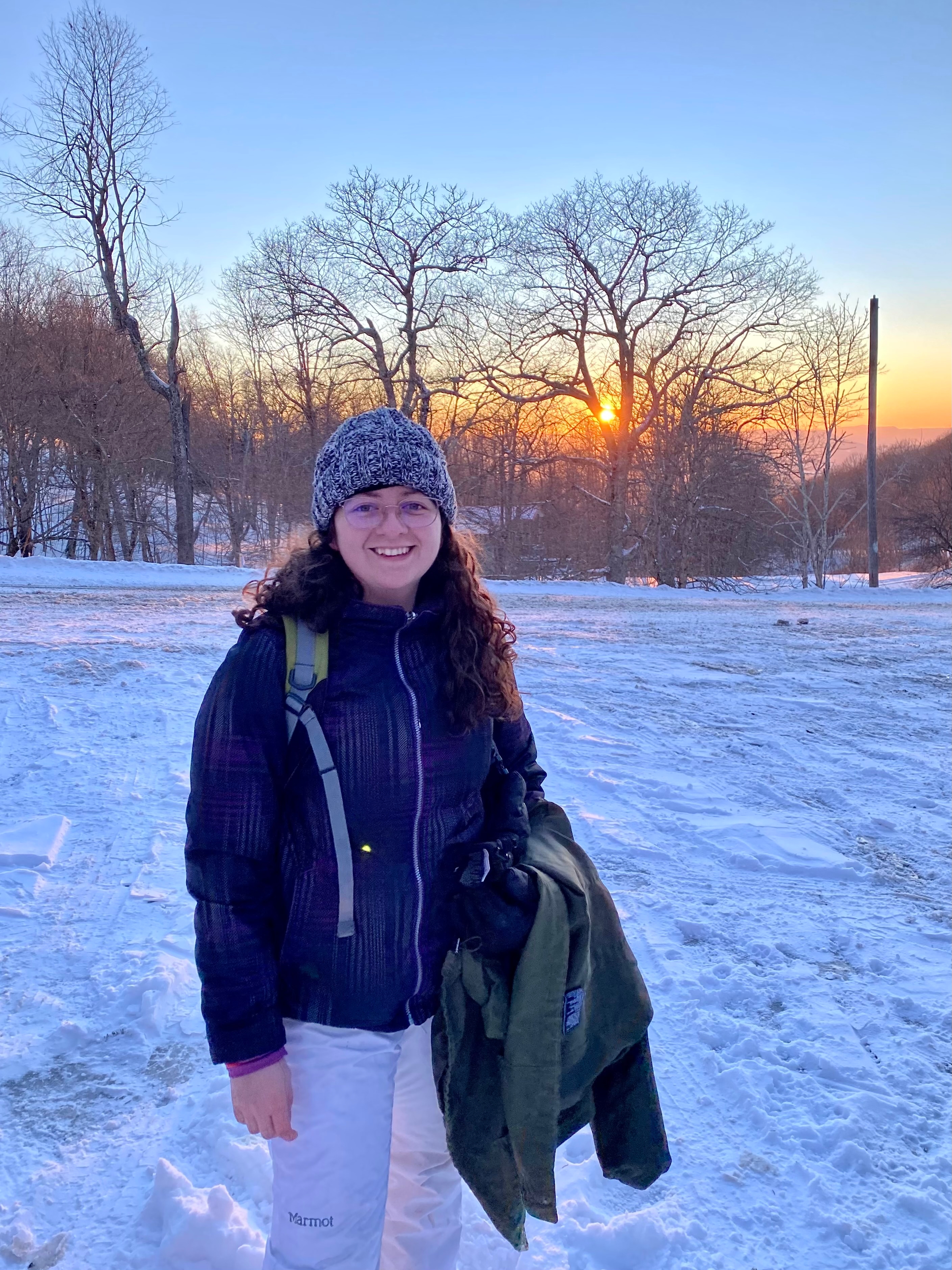
Mariana Castaneda-Guzman (she/her/hers) is a Ph.D. student at Virginia Tech in the Department of Fish and Wildlife Conservation, working under the guidance of Dr. Paul Angermeier and Dr. Emmanuel Frimpong. Her main interests are data analytics, including remote sensing and big data analysis, to inform and optimize strategic decisions related to ecosystem health and climate change adaptation in the private and public sectors. Mainly by applying modeling tools to reveal patterns from ecological data and developing simulations to test “what-if” scenarios of landscape and climate change. Her undergraduate degree in systems engineering has given her a sound basis in mathematics, programming, and statistics. Her master’s research, working alongside Dr. Luis E. Escobar in the Department of Fish and Wildlife Conservation at Virginia Tech, allowed her to apply her engineering skills to problems of wildlife diseases and ecosystem dynamics under diverse climate change conditions using novel machine learning methods.
By linking and collaborating with public and academic institutions via big-data aquatic ecosystem health studies, she plans to fill a gap generally neglected and where women researchers are rare. Studies in terrestrial ecosystems reveal many risks of climatic variation to human, livestock, and wildlife health. Nevertheless, studies of climate change effects on aquatic ecosystems lag behind. Freshwater fishes play vital roles in aquatic ecosystems’ health. They serve as food sources for many animals and human communities and are culturally and economically important across the globe. She plans to study how climate and land use change could affect range-wide distributions and, ultimately persistence, of selected freshwater fishes. This research will account for historical, present, and future climatic and land use conditions in aquatic systems using a big-data approach to implement novel machine-learning ecological niche modeling based on satellite-derived and other existing datasets. Developing better tools to reconstruct the potential geographic distributions of data-limited species can improve management and policy efficacy, leading to greater societal benefits. This research is of primary interest to professionals working on conserving and managing aquatic fauna, fisheries and seafood industries, and public health concerning foodborne diseases.